Revolutionizing Efficiency: The Automatic Image Annotation Tool in Software Development
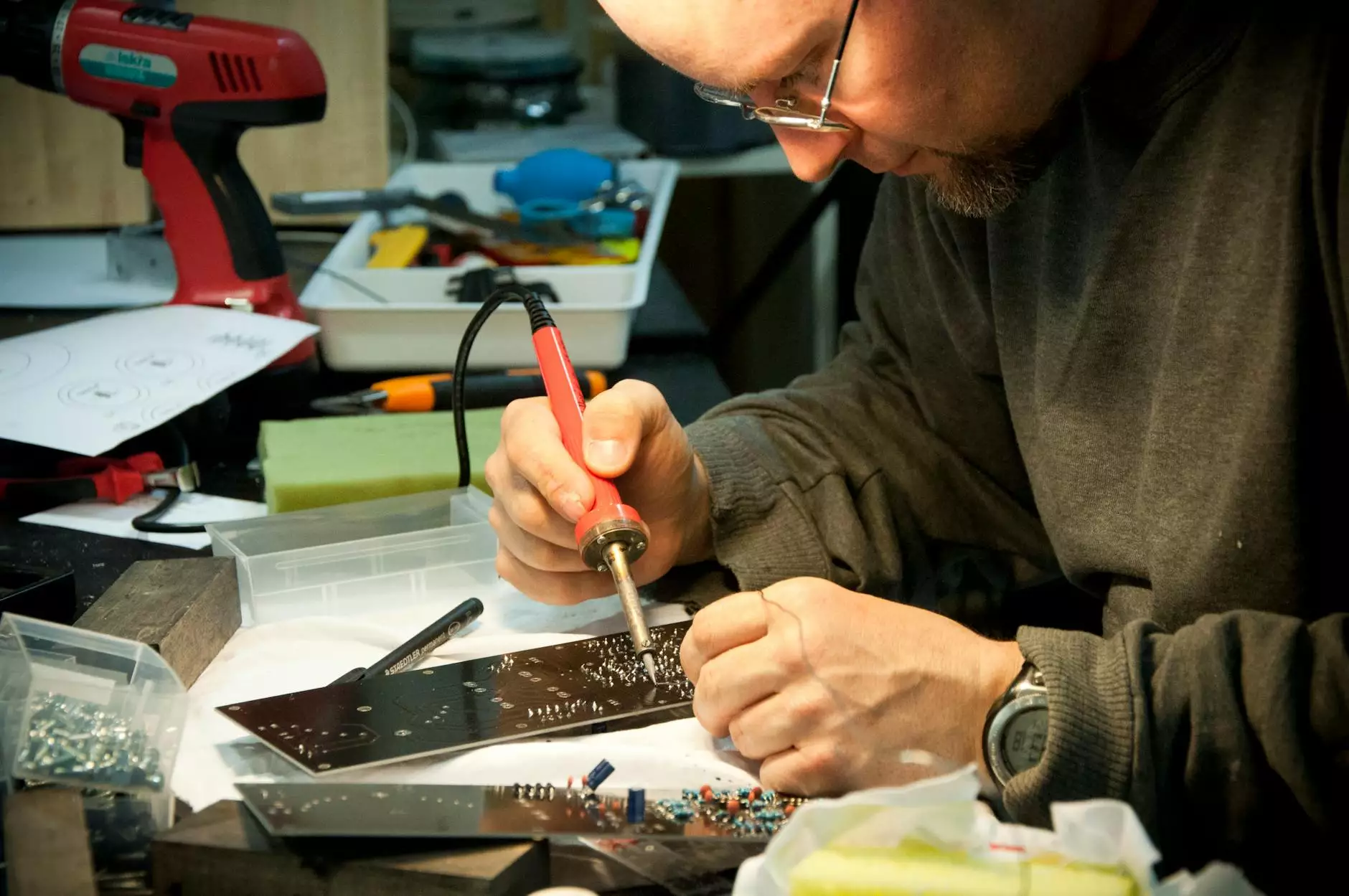
In the digital age, where efficiency and accuracy are paramount, the role of technology in streamlining processes cannot be overstated. One such technology that is making waves in the realm of software development is the automatic image annotation tool. This remarkable tool not only saves time but also enhances the quality of data annotation, making it easier for developers to create and train machine learning models.
Understanding the Concept of Image Annotation
Image annotation is the process of labeling images with relevant tags and metadata, enabling machines to understand the visual content. This process is crucial in various industries, especially in:
- Artificial Intelligence (AI) - Training AI models to recognize specific objects or patterns.
- Machine Learning (ML) - Providing labeled data sets for supervised learning techniques.
- Computer Vision - Enabling computers to interpret and make decisions based on visual data.
The Importance of Automatic Image Annotation Tools
The emergence of automatic image annotation tools has transformed the landscape of data preparation for AI and ML projects. Here’s why they are essential:
1. Efficiency and Speed
Automatic image annotation tools significantly reduce the time required for manual annotation of images. For businesses aiming to train their models quickly, these tools offer a substantial advantage. Instead of spending countless hours on labor-intensive tasks, developers can rely on automated solutions to handle large datasets with ease.
2. Cost-Effectiveness
Utilizing an automatic image annotation tool can lead to significant cost savings. By minimizing labor costs associated with manual annotation, businesses can allocate resources more effectively towards innovation and development. This is particularly beneficial for startups and small enterprises aiming to maximize their budgets.
3. Accuracy and Consistency
One of the challenges of manual annotation is the potential for human error. Automatic annotation tools, on the other hand, utilize advanced algorithms to ensure a higher degree of accuracy and consistency. This is critical as the quality of the training data directly impacts the performance of machine learning models.
How Does an Automatic Image Annotation Tool Work?
The functionality of automatic image annotation tools is rooted in advanced technologies including:
- Machine Learning Algorithms - These algorithms learn from pre-labeled datasets to recognize patterns and features in images.
- Deep Learning Techniques - Leveraging neural networks, these tools can identify complex objects and scenes in images with high precision.
- Natural Language Processing (NLP) - Enabling the generation of descriptive tags and metadata based on the content of images.
The Role of Automatic Image Annotation Tools in Software Development
In the realm of software development, particularly when creating applications that leverage computer vision, the use of automatic image annotation tools can lead to remarkable advancements. Here are some areas where these tools shine:
1. Accelerating Development Cycles
With the rapid evolution of technology, software development cycles have shortened significantly. An automatic image annotation tool can expedite the data preparation phase, enabling developers to focus on building and deploying applications more effectively.
2. Enhancing Collaboration
Development teams, often comprising data scientists, software engineers, and domain experts, require seamless collaboration. Automatic annotation tools provide a centralized platform where annotated data can be shared, reviewed, and refined, ensuring all team members work with the same high-quality datasets.
3. Improving Model Performance
The accuracy of machine learning models is heavily dependent on the quality of the training data. By using automatic image annotation tools, developers can ensure that their datasets are not only large but also accurately labeled. This leads to better-performing models that can deliver real-world results.
Choosing the Right Automatic Image Annotation Tool
With numerous tools available in the market, selecting the right automatic image annotation tool for your business needs can be daunting. Here are factors to consider:
1. User-Friendliness
The tool should have an intuitive interface that allows users to easily upload images, manage annotations, and retrieve labeled data without requiring extensive training.
2. Customization Options
Look for a tool that provides customization options to suit specific project requirements, such as the ability to adjust labeling criteria or set up unique workflows.
3. Integration Capabilities
The chosen tool should seamlessly integrate with existing software development processes. Compatibility with deployment environments and data management systems is crucial.
4. Algorithm Accuracy
Research the algorithms used by the tool to ensure they align with your accuracy requirements. Some tools may specialize in certain types of images or topics, which can impact their overall effectiveness.
5. Support and Community
Consider the support and community engagement around the tool. Comprehensive documentation, customer support, and an active user community can significantly ease the implementation and troubleshooting processes.
Case Studies: Success Stories Using Automatic Image Annotation Tools
To illustrate the effectiveness of automatic image annotation tools, let’s review a few successful case studies:
1. Retail and E-commerce
A major e-commerce retailer utilized automatic image annotation to enhance their product image search capabilities. By effectively tagging and categorizing millions of product images, they improved their search functionality, leading to a notable increase in sales.
2. Healthcare
In the healthcare sector, a company leveraged automatic image annotation tools to develop a diagnostic application that analyzes medical images. This not only improved diagnostic accuracy but also reduced the turnaround time for patient results, enhancing overall patient care.
3. Autonomous Vehicles
Self-driving car manufacturers employ automatic image annotation tools to label vast datasets comprising street images. Accurate annotations help in training their AI models to better understand real-world driving conditions, enhancing safety and reliability.
The Future of Automatic Image Annotation
The landscape of software development continues to evolve, and the future of automatic image annotation tools is promising. As machine learning and AI technologies advance, we can expect:
1. Improved Accuracy and Speed
Future iterations of these tools will likely incorporate even more sophisticated algorithms that enhance both speed and accuracy, making the annotation process more efficient than ever before.
2. Broader Application Domains
As industries beyond tech begin to adopt AI solutions, the demand for image annotation tools is set to grow across various fields, including agriculture, security, and finance.
3. Integration with Augmented and Virtual Reality
With the rise of augmented and virtual reality applications, automatic annotation tools may integrate capabilities to handle 3D models and environments, further expanding their utility.
Conclusion
The adoption of an automatic image annotation tool is not just a trend, but a strategic move that can redefine the way businesses approach software development. By embracing this technology, organizations can unlock the potential of their data, streamline workflows, and position themselves competitively in an increasingly data-driven world.
At Keymakr, we believe that innovation through automation is the key to unlocking efficiency in software development processes. Explore how our solutions can empower your organization today!