Understanding Labeling Tools for Machine Learning
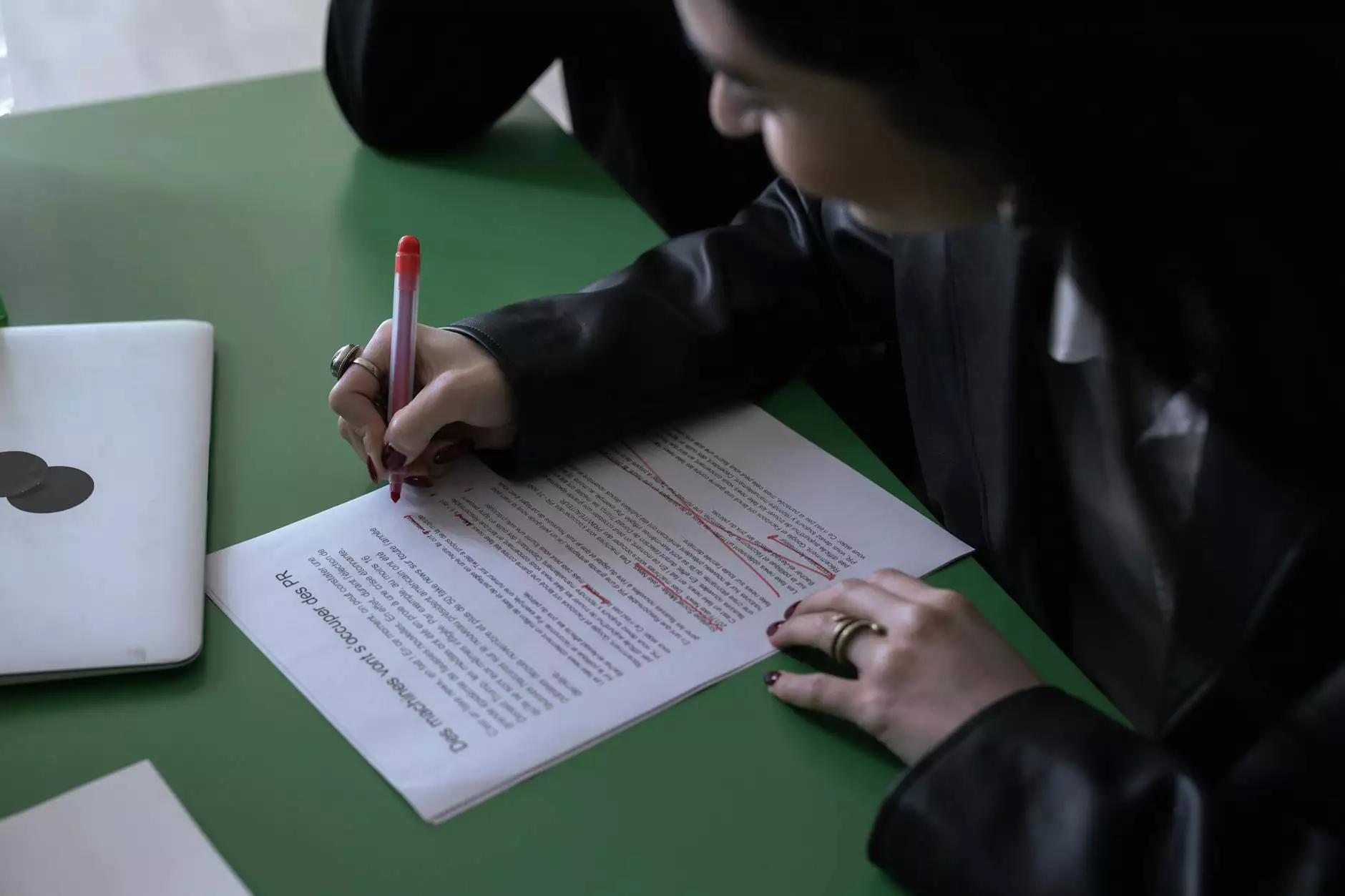
In the evolving landscape of artificial intelligence and machine learning, the significance of labeling tools for machine learning cannot be overstated. As organizations increasingly rely on data-driven models, the quality and accuracy of the annotated data become pivotal for success. This article delves into the intricacies of labeling tools, their importance, and how they facilitate efficient data annotation processes.
What Are Labeling Tools for Machine Learning?
Labeling tools for machine learning are specialized software applications designed to aid in the data annotation process. These tools allow users to annotate data sets, which typically include images, text, audio, and video, by adding metadata, tags, or labels to the raw data. This transformation of raw data into annotated datasets enables machine learning models to learn, recognize patterns, and make informed decisions.
The Importance of Data Annotation in Machine Learning
Data annotation is a critical step in the machine learning pipeline. Here’s why:
- Quality of Data: The accuracy of a machine learning model directly correlates with the quality of the annotated data. High-quality annotations lead to better performance and reliable predictions.
- Supervised Learning: Most machine learning models require labeled data to train algorithms. Supervised learning uses labeled datasets to teach models to function effectively.
- Faster Development Cycles: Efficient data annotation accelerates the development process of machine learning applications, allowing organizations to deploy solutions more quickly.
Types of Labeling Tools for Machine Learning
Labeling tools can vary significantly in terms of functionality and application. Here are some common types:
1. Image Annotation Tools
These tools facilitate the annotation of images and visual data. Common features include:
- Bounding Boxes: Used for object detection, allowing users to draw boxes around objects in images.
- Polygon Annotation: More precise than bounding boxes, users can create complex shapes to capture object outlines.
- Semantic Segmentation: Divides images into segments, enabling pixel-level annotations.
2. Text Annotation Tools
Text annotation tools help in categorizing and labeling textual data. Key features include:
- Named Entity Recognition: Identifies entities within text, such as names, dates, and locations.
- Sentiment Annotation: Assesses the sentiment expressed in text, important for natural language processing (NLP) applications.
- Part of Speech Tagging: Labels parts of speech in sentences, aiding in syntactic analysis.
3. Audio and Video Annotation Tools
These tools are essential for annotating audio clips and video files. They often feature:
- Speech Recognition: Converts spoken words into written text for further analysis.
- Action Recognition: Annotates movements and actions occurring in video content.
- Audiovisual Synchronization: Synchronizes audio and video annotations for multi-modal datasets.
Key Features of Effective Labeling Tools
When assessing labeling tools for machine learning, consider the following features:
- Usability: An intuitive user interface that makes it easy for users to navigate through the annotation process.
- Collaboration: Tools that allow multiple users to collaborate and annotate data simultaneously enhance productivity and efficiency.
- Integration: Compatibility with other data processing and machine learning platforms is vital for seamless workflows.
- Scalability: The ability to handle large datasets as organizations grow is essential for sustainable operations.
- Quality Control: Tools that include functionalities for reviewing and validating annotations improve overall data quality.
Advantages of Using Keylabs.ai for Data Annotation
Keylabs.ai offers a robust data annotation platform that stands out for its user-centric approach and cutting-edge features. Here's why organizations should consider Keylabs.ai for their annotation needs:
1. Comprehensive Data Annotation Solutions
Keylabs.ai provides tailored solutions for a variety of data types, allowing businesses to aggregate all their annotation tasks under one roof. From image to text and audiovisual data, our platform supports multiple annotation formats, enabling versatility.
2. Enhanced Collaboration Features
With tools designed for real-time collaboration, teams can work together seamlessly, minimizing errors and maximizing productivity. The ability to assign tasks and monitor progress ensures that all team members are aligned on project goals.
3. AI-Powered Assistances
Our AI-driven features assist annotators by suggesting labels, thereby expediting the labeling process and ensuring consistency across annotations. This not only saves time but also enhances the quality of the output data.
4. Secure and Scalable Infrastructure
Data security is paramount at Keylabs.ai. Our platform is built on robust security protocols to protect sensitive data, ensuring that you can focus on achieving your goals without compromising on safety. Additionally, our infrastructure can scale seamlessly with your growing data requirements.
Implementing a Successful Data Annotation Strategy
To successfully integrate labeling tools for machine learning into your workflow, consider the following strategic steps:
1. Define Clear Objectives
Establish what you aim to achieve with your data annotation project. Setting clear goals helps in selecting the right tools and measuring success.
2. Choose the Right Labeling Tools
After assessing your requirements, choose a labeling tool that aligns with your objectives. Consider factors like usability, scalability, and integrations.
3. Train Your Annotation Team
Ensure that your team of annotators is well-trained to utilize the chosen tools effectively. Provide them with comprehensive guidelines and best practices for data annotation.
4. Monitor and Review The Annotation Process
Regularly evaluate the quality of annotations. Utilize tools that offer quality control features to catch errors early and refine your processes.
5. Iterate and Improve
Data annotation is often an iterative process. Use feedback and performance metrics to continually enhance your annotation strategies and tooling choices.
The Future of Labeling Tools for Machine Learning
As machine learning continues to advance, the demand for sophisticated labeling tools will grow. Future trends may include:
- Increased Automation: The use of AI to automate part of the annotation process is on the rise, helping to reduce time and labor costs.
- Enhanced AI Integration: More labeling tools will harness machine learning capabilities to improve their own functionality, making annotations faster and more accurate.
- Real-Time Collaboration Advances: As remote work becomes the norm, the need for tools that support real-time collaboration will become even more critical.
Conclusion
In the realm of machine learning, the significance of effective labeling tools for machine learning cannot be underestimated. They are essential for transforming raw data into high-quality annotated datasets, which are crucial for building robust models. By leveraging platforms like Keylabs.ai, organizations can enhance their data annotation efforts, ensuring they remain competitive in a data-driven world. Keep an eye on the evolving landscape, as innovative solutions continue to emerge to meet the growing needs of this dynamic field.
Explore more about our products and offerings at Keylabs.ai.